On June 2024, I received the Ph.D. degree in the College of Information Science & Electronic Engineering (ISEE) at Zhejiang University, Hangzhou, China, where I am jointly advised by Prof. Zhiguo Shi (Vice dean of the College of ISEE, IEEE Fellow), Prof. Quan Yu (Academician of the Chinese Academy of Engineering), and Prof. Chengwei Zhou. From Oct. 2022 to Oct. 2023, I was a joint training Ph.D. student in the Department of Acoustics & Signal Processing at Aalto University, Espoo, Finland, where I am supervised by Prof. Sergiy Vorobyov (IEEE Fellow).
My research interests include array signal processing, tensor signal processing, and AI for signal processing. To effectively process large-scale high-dimensional array signals in future communication and sensing systems, I am working on the theories and techniques of sub-Nyquist tensor processing, efficient tensor optimization, and resource-efficient tensorized neural networks. The proposed methods facilitate direction-of-arrival (DOA) estimation, parameter estimation, and adaptive beamforming with low system costs and competitive performance, laying the foundations for ISAC, target localization, and anti-interference.
I have published 30+ papers at top journals and international conferences with google citations .
I am an active reviewer for IEEE TSP, IEEE TAES, IEEE TVT, IEEE SPL, Signal Processing, and IET Signal processing.
🔥 News
- 2024.11: 🎉 Our paper Coarray tensor direction-of-arrival estimation is recognized as an ESI Highly Cited Paper.
- 2024.08: 🎉 Our paper Deep tensor 2-D DOA estimation for URA is accepted to IEEE Transactions on Signal Processing
- 2023.11: 🎉 Our paper received the Excellent Scientific Papers from China Association for Science and Technology
- 2023.08: 🎉 Our paper Sub-Nyquist tensor beamformer: A coprimality constrained design is accepted to IEEE Transactions on Signal Processing
- 2023.06: 🎉 Our paper Tensorized neural layer decomposition for 2-D DOA estimation is recognized as the Top 3% paper of all accepted papers at IEEE ICASSP 2023
- 2023.03: 🎉 Our papers Coarray tensor direction-of-arrival estimation and Coarray tensor completion for DOA estimation are respectively accepted to IEEE Transactions on Signal Processing and IEEE Transactions on Aerospace and Electronic Systems
📝 Publications
Journal papers
-
H. Zheng, Z. Shi, C. Zhou, S. A. Vorobyov, and Y. Gu, “Deep tensor 2-D DOA estimation for URA,” IEEE Trans. Signal Process., vol. 72, pp. 4065-4080, 2024.
-
H. Zheng, C. Zhou, Z. Shi, Y. Gu, and Y. D. Zhang, “Coarray tensor direction-of-arrival estimation,” IEEE Trans. Signal Process., vol. 71, pp. 1128-1142, 2023.
-
H. Zheng, C. Zhou, Z. Shi, and G. Liao, “Sub-Nyquist tensor beamformer: A coprimality constrained design,” IEEE Trans. Signal Process., vol. 71, pp. 4163-4177, 2023.
-
H. Zheng, Z. Shi, C. Zhou, and A. L. F. de Almeida, “Coarray tensor completion for DOA estimation,” IEEE Trans. Aerosp. Electron. Syst., vol. 59, no. 5, pp. 5472-5486, Oct. 2023.
-
H. Zheng, C. Zhou, S. Vorobyov, Q. Wang, and Z. Shi, “Decomposed CNN for sub-Nyquist tensor-based 2-D DOA estimation,” IEEE Signal Process. Lett., vol. 30, pp. 708-712, 2023.
-
H. Zheng, C. Zhou, Z. Shi, and A. L. F. de Almeida, “SubTTD: DOA estimation via sub-Nyquist tensor train decomposition,” IEEE Signal Process. Lett., vol. 29, pp. 1978-1982, 2022.
-
H. Zheng, C. Zhou, Z. Shi, and Y. Gu, “Structured tensor reconstruction for coherent DOA estimation,” IEEE Signal Process. Lett., vol. 29, pp. 1634-1638, 2022.
-
H. Zheng, Z. Shi, C. Zhou, M. Haardt, and J. Chen, “Coupled coarray tensor CPD for DOA estimation with coprime L-shaped array,” IEEE Signal Process. Lett., vol. 28, pp. 1545-1549, July 2021.
-
L. Lin, J. Tong, H. Lin, H. Zheng and Z. Shi, “Joint Target Localization and Channel Estimation for ODDM-ISAC Systems,” IEEE Signal Processing Letters, 2025.
-
Y. Yue, H. Zheng, Z. Shi and G. Liao, “Two-stage Reconstruction for Co-array 2D DOA Estimation of Mixed Circular and Noncircular Signals,” IEEE Transactions on Vehicular Technology, 2025.
-
Y. Fan, Y. Wang, H. Zheng and Z. Shi, “Video2mmPoint: Synthesizing mmWave Point Cloud Data from Videos for Gait Recognition,” IEEE Sensors Journal, 2024.
-
F. Xu, H. Zheng, and S. A. Vorobyov, “Tensor-based 2D DOA estimation for L-shaped nested array,” IEEE Trans. Aerosp. Electron. Syst., vol. 60, no. 1, pp. 604-618, 2024.
-
L. Lin, H. Zheng, C. Zhou, S. He, and Z. Shi, “Nonorthogonal waveform assisted DOA estimation for joint MIMO sensing and communication,” EURASIP J. Adv. Signal Process., vol. 2023, no. 1, Art. no. 22, Feb. 2023.
-
B. Li, F. Cheng, H. Zheng *, Z. Shi, and C. Zhou, “Structural-missing tensor completion for robust DOA estimation with sensor failure,” Appl. Sci., vol. 13, no. 23, Art. no. 12740, 2023. (*corresponding author)
中文期刊论文
-
郑航, 史治国, 王勇, 周成伟, “面向结构化稀疏感知的张量阵列信号处理,” 《电子学报》, 2025.
-
郑航, 周成伟, 王勇, 史治国, “EMVS互质面阵张量波束成形,”《信号处理》, 40(2): 250-262, 2024.
-
周成伟, 郑航, 顾宇杰, 王勇, 史治国, “互质阵列信号处理研究进展:波达方向估计与自适应波束成形,” 《雷达学报》, 8(5): 558-577, 2019.
Conference papers
-
H. Zheng, C. Zhou, S. A. Vorobyov, and Z. Shi, “Tensorized neural layer decomposition for 2-D DOA estimation,” in Proc. IEEE Int. Conf. Acoust., Speech, Signal Process. (ICASSP), Rhodes Island, Greece, June 2023. (Top 3% paper of all accepted papers)
-
H. Zheng, C. Zhou, A. L. F. de Almeida, Y. Gu, and Z. Shi, “DOA estimation via coarray tensor completion with missing slices,” in Proc. IEEE Int. Conf. Acoust., Speech, Signal Process. (ICASSP), Singapore, May 2022, pp. 5053-5057.
-
H. Zheng, C. Zhou, Z. Shi, and C. Yan, “Joint coprime weights optimization for sub-Nyquist tensor beamforming,” in Proc. IEEE Radar Conf., New York, USA, Mar. 2022, pp. 1-6.
-
H. Zheng, C. Zhou, Y. Gu, and Z. Shi, “Two-dimensional DOA estimation for coprime planar array: A coarray tensor-based solution,” in Proc. IEEE Int. Conf. Acoust., Speech, Signal Process. (ICASSP), Barcelona, Spain, May 2020, pp. 4562-4566.
-
H. Zheng, C. Zhou, Y. Wang, J. Zhou, and Z. Shi, “Sample fourth-order cumulant tensor denoising for DOA estimation with coprime L-shaped array,” in Proc. Asilomar Conf. Signals, Syst., Comput., Pacific Grove, CA, Nov. 2021, pp. 1668-1672.
-
H. Zheng, C. Zhou, Y. Wang, and Z. Shi, “2-D DOA estimation for coprime cubic array: A cross-correlation tensor perspective,” in Proc. Int. Symp. Antennas & Propag. (ISAP), Osaka, Japan, Jan. 2021, pp. 447-448. (Best Paper Award)
-
Y. Wang, H. Zheng, C. Zhou, C. Yan, and Z. Shi, “Joint DOD and DOA estimation via coupled CPD with sparse MIMO radar for IIoT,” in Proc. CIE Int. Conf. Radar, Haikou, China, Dec. 2021, pp. 1566-1570.
-
F. Cheng, H. Zheng, Z. Shi, and C. Zhou, “Fiber-missing tensor completion for DOA estimation with sensor failure,” in Proc. Int. Conf. Autonomous Unmanned Systems, Xian, China, Sep. 2022, pp. 3457-3466. (Best Paper Award)
-
L. Lin, Z. Zhang, H. Zheng, C. Zhou, and Z. Shi, “DOA estimation for joint sensing and communication with nonorthogonal waveform,” in Proc. Asilomar Conf. Signals, Syst., Comput., Pacific Grove, CA, Nov. 2022, pp. 298-302.
-
L. Lin, H. Zheng, C. Zhou, and Z. Shi, “Channel covariance matrix construction for DOA estimation with limited communication symbols,” in Proc. IEEE Radar Conf., TX, USA, May 2023, pp. 1-5.
-
C. He, H. Zheng, B. Li, C. Zhou, and Z. Shi, “DOA estimation via meta-learning under array sensor failures,” in Proc. Int. Conf. Radar, Sydney, Australia, Nov. 2023.
-
F. Cheng, H. Zheng, Z. Shi, and C. Zhou, “Tensorial Hankel reconstruction for coherent DOA estimation with sensor failure,” in Proc. IEEE Int. Conf. Commun. Technol. (ICCT), Wuxi, China, Oct. 2023, pp. 221-225.
-
L. Lin, H. Zheng, S. A. Vorobyov, C. Zhou, and Z. Shi, “Sensing-aided communication channel estimation with tensor-based moving target localization,” in Proc. IEEE Int. Conf. Acoust., Speech, Signal Process. (ICASSP), Seoul, Korea, Apr. 2024, pp. 8606-8610.
-
Y. Ren, H. Zheng and Z. Shi, “Tensor-Based Subspace Fusion for Direct Position Determination,” in Proc. IEEE/CIC International Conference on Communications in China (ICCC), Hangzhou, China, 2024, pp. 208-212.
-
F. Qian, H. Zheng, C. Zhou and Z. Shi, “Spatial Sectorized Neural Network for 2-D DOA Estimation in the Full Azimuth,” in Proc. European Signal Processing Conference (EUSIPCO), Lyon, France, 2024, pp. 1147-1151.
📄 Patents
- H. Zheng, C. Zhou, Z. Shi, and J. Chen. Method for estimation direction of arrival of an L-type coprime array based on coupled tensor decomposition, US 12117545, 2024-10-15.
- C. Zhou, H. Zheng, J. Chen, Z. Shi. High resolution, accurate, two-dimensional direction-of-arrival estimation method based on coarray tensor spatial spectrum searching with coprime planar array, US 11300648, 2022-04-12.
- Z. Shi, H. Zheng, C. Zhou, J. Chen. Two-dimensional direction-of-arrival estimation method for coprime planar array based on structured coarray tensor processing, US 11408960, 2022-08-09.
- J. Chen, H. Zheng, Z. Shi, C. Zhou. Spatial spectrum estimation method with enhanced degree-of-freedom based on block sampling tensor construction for coprime planar array, US 11422177, 2022-08-23.
- Z. Shi, H. Zheng, C. Zhou, J. Chen, Y. Wang. Three-dimensional co-prime cubic array direction-of-arrival estimation method based on a cross-correlation tensor, US 11879986, 2024-01-23.
- 史治国, 郑航, 周成伟, 陈积明, 王勇. 基于互相关张量的三维互质立方阵列波达方向估计方法, ZL 202110065604.4, 2022-02-18.
- 郑航, 周成伟,颜成钢,陈剑,史治国,陈积明. 基于耦合张量分解的 L 型互质阵列波达方向估计方法, ZL 202110781692.8, 2022-03-22.
- 郑航, 周成伟,颜成钢,史治国,王勇,陈积明. 基于结构化虚拟域张量信号处理的互质面阵二维波达方向估计方法, ZL 202010370072.0, 2022-09-20.
- 郑航, 王勇,周成伟,颜成钢,史治国,陈积明. 基于平面互质阵列块采样张量信号构造的自由度增强型空间谱估计方法, ZL 202010370913.8, 2022-10-04.
- 郑航, 周成伟,史治国, 王勇,陈积明. 基于平面互质阵列虚拟域张量空间谱搜索的高分辨精确二维波达方向估计方法, ZL 202010371305.9, 2022-12-27.
- 郑航, 史治国, 陈积明, 颜成钢, 周成伟, 周金芳. 基于最小化准则的电磁矢量互质面阵张量功率谱估计方法, ZL 202011499625.9, 2023-06-27.
- 史治国, 郑航, 陈积明, 颜成钢, 周成伟, 王勇. 基于乘性张量波束扫描的电磁矢量互质面阵多维参数估计方法, ZL 202011490509.0, 2023-06-27.
- 史治国, 陈剑锋, 单国锋, 郑航. 一种自动探测测向无人机的方法, ZL 202010786192.9, 2023-07-07.
- J. Chen, Z. Shi, H. Zheng, C. Zhou. Composite tensor beamforming method for electromagnetic vector coprime planar array, US 11841448, 2023-12-12.
🎖 Honors and Awards
- 2025.01:中国电子教育学会优秀博士学位论文
- 2023.11: Excellent Scientific Papers from China Association for Science and Technology(中国科协优秀科技论文)
- 2023.06: IEEE ICASSP 2023 Top 3% of all accepted papers
- 2022.09: ICAUS 2022 Best paper award
- 2020.12: IEICE ISAP 2020 Best paper award
- 2023.10:National Scholarship for Graduate Excellence(研究生国家奖学金)
- 2021.12: Chu Kochen Scholarship(浙江大学研究生竺可桢奖学金)
- 2023.05, 2021.06: 华为菁英奖学金
- Supported by the China Scholarship Council (CSC) for joint training at Aalto University
- 全国数学建模竞赛上海市一等奖, 全国二等奖
- “创青春”上海市大学生创业大赛创业计划类金奖
- “挑战杯”全国大学生创业大赛银奖
- “互联网+”大学生创新创业大赛上海市铜奖
📖 Educations
- 2019.09 - 2024.06, Ph.D. student, College of Information Science & Electronic Engineering, Zhejiang University, Hangzhou, China
- 2022.10 - 2023.10, Joint training Ph.D. student, Department of Acoustics & Signal Processing, Aalto University, Espoo, Finland
- 2015.09 - 2019.07, Bachelor, College of Transportation Engineering, Tongji University, Shanghai, China
💬 Research Highlights
Sub-Nyquist tensor DOA estimation
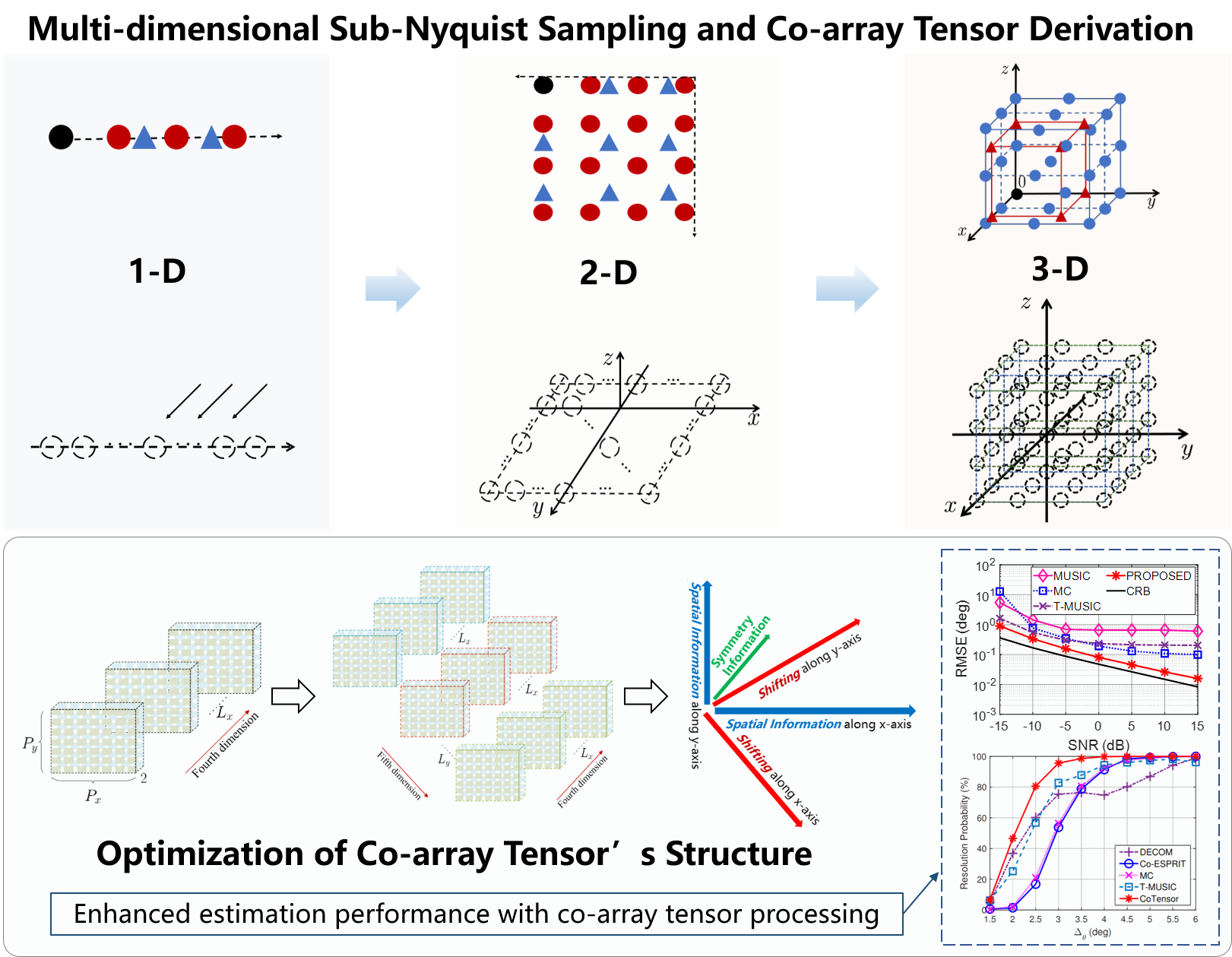
- Derive the high-order co-array tensor from sub-Nyquist tensor signals, and achieve co-array tensor-based direction-of-arrival estimation with high accuracy, super resolution and increased degrees-of-freedom
- Optimize the uniqueness condition of co-array tensor decomposition, and identify multiple sources that exceed the number of physical array sensors
- Complete the coarray tensor with missing slices to exploit the full co-array information
Analytical and numerical results are presented in our papers published in IEEE TSP, IEEE TAES, and IEEE ICASSP.
Sub-Nyquist tensor beamforming
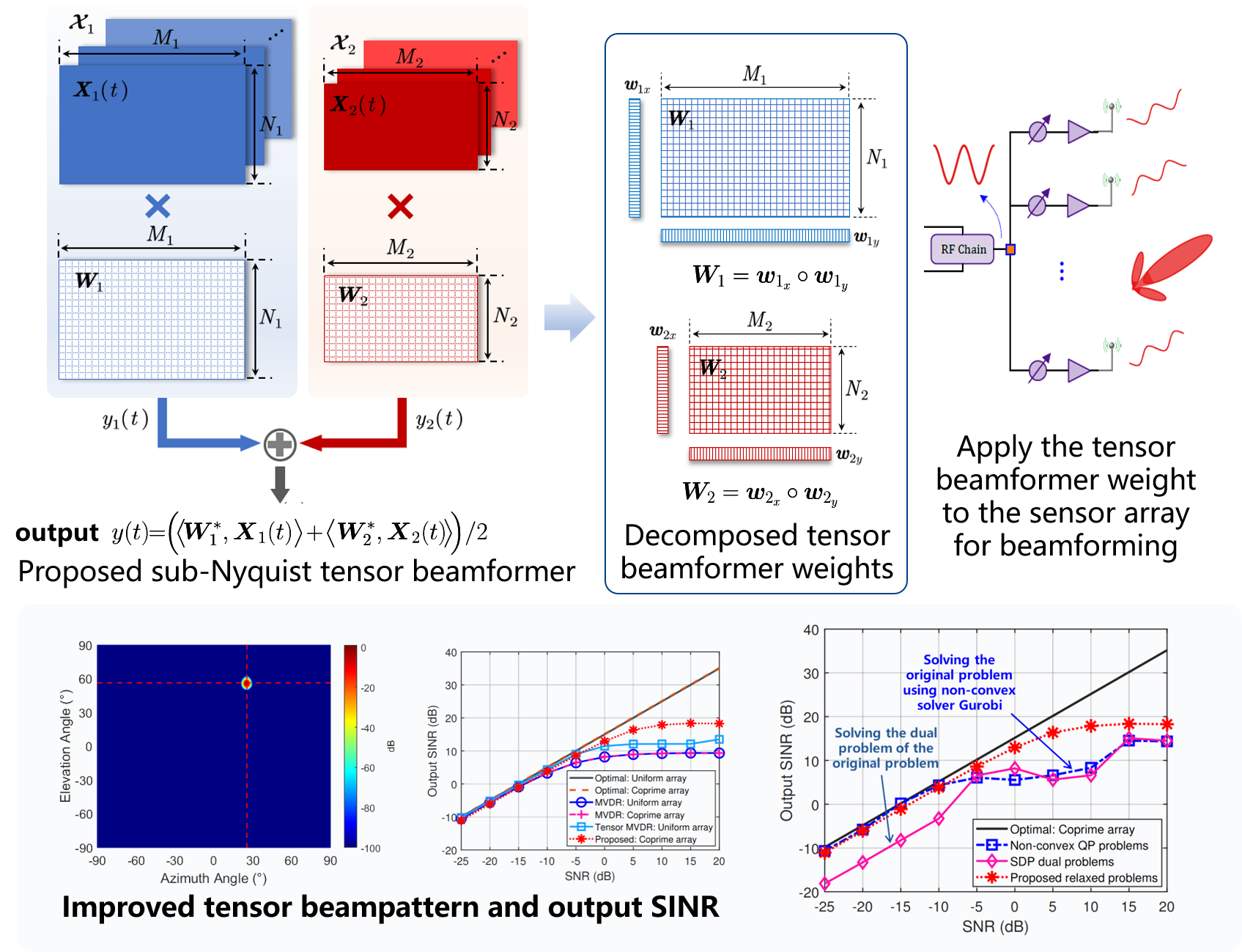
- Develop the principle of tensor signal filtering with sparse array, thereby enhancing the signal-of-interest with reduced system burden
- Enhance mainlobe while suppressing sidelobes in the beampattern by solving tensor beamformer weight optimization problems
- Improve output signal-to-interference-plus-noise ratio (SINR) for strong anti-interference
The algorithm for beamforming design and results are presented in our papers published on IEEE TSP and Radarconf.
Tensorized neural networks for signal processing
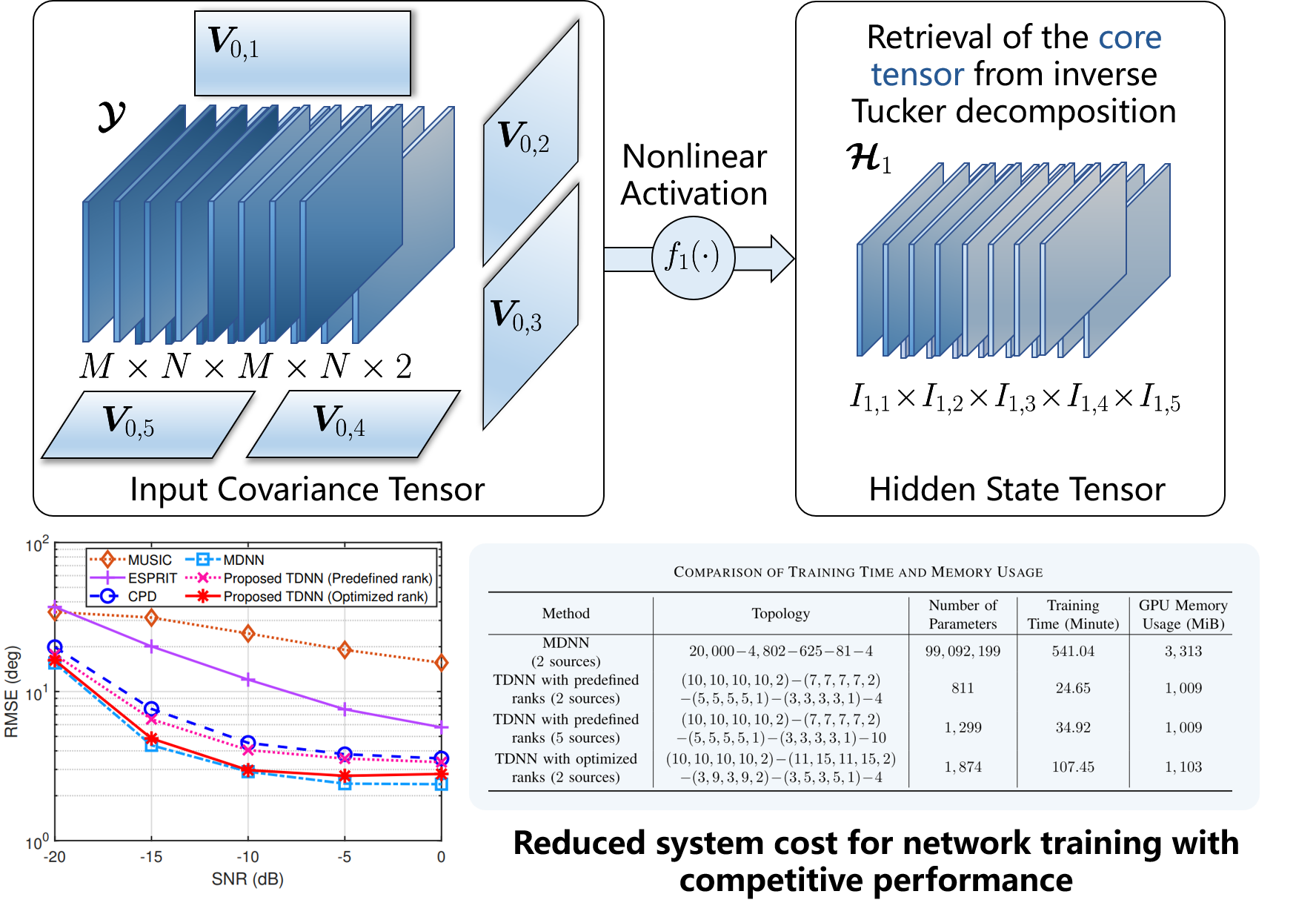
- Design tensorized neural networks to efficiently learn inherent features from multi-dimensional array signals, leading to robust performance in non-ideal situations such as low signal-to-noise (SNR) ratio and limited snapshots
- Highly compress the parameters in tensorized neural layers, and accelerate the training speed of the tensorized neural network
This work was presented at IEEE ICASSP 2023, and recognized as the Top 3% paper. The extended work is published on IEEE TSP.